AI is making significant strides across various industries, and healthcare is no exception. From improving diagnostic accuracy to easing administrative burdens, AI is ready to transform the way healthcare is delivered and experienced. This blog post is the combination of research for our Podcast Dcypher and grounded insights from the builders in the space we actually interviewed, Nate Verhagen and Leonard Rinser.
The Current State of AI in Healthcare
Reducing Administrative Workloads
Administrative tasks are a major source of burnout for clinicians. AI technologies, such as ambient clinical documentation tools, are helping to alleviate this burden. One study showed that physicians spend two-thirds of their time talking to patients, but a quarter of that was talking to the patient while typing. Another survey showed that some physicians spend almost 20 hours a week on paperwork and documentation
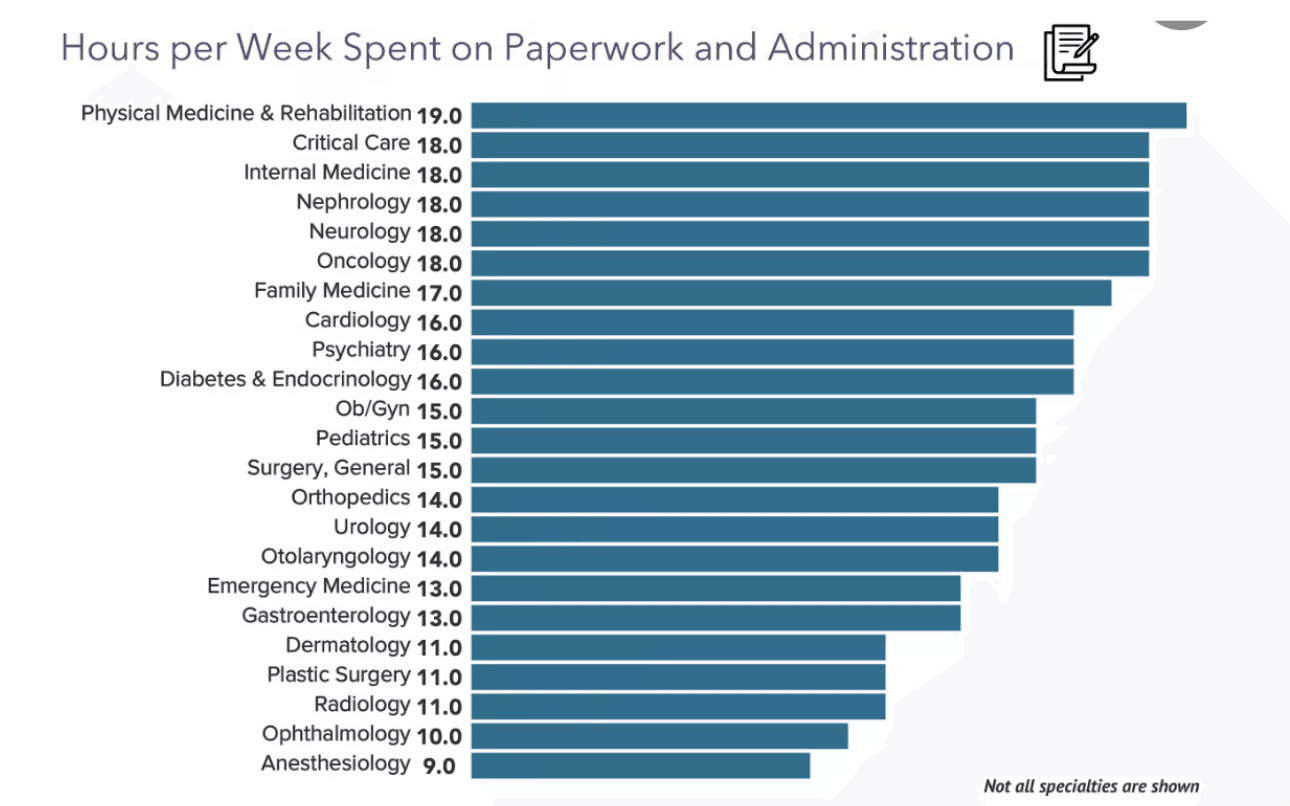
These AI tools can automatically transcribe and organize notes from physician-patient interactions, allowing doctors to focus more on patient care. This shift not only improves efficiency but also enhances the patient experience by allowing for more face-to-face interaction.
In the past year, the FDA approved 171 AI or machine learning devices for use in medicine, with a projected 30% increase in approvals for 2024. Startups like Corti and Nabla are eliminating doctors' time spent on documentation by providing instant clinical notes, updating medical records, and analyzing content. This allows clinicians to focus on the human aspects of care, a view echoed by Nate Verhagen.
Enhancing Decision-Making
Physicians can now access vast medical research, clinical guidelines, and trials, continuously updated and rooted in evidence-based practices. Tools like OpenEvidence and Consensus democratize access to medical literature, transforming traditional methods of navigating medical information.
AI-augmented decision-making is becoming the norm. Real-time AI co-pilots, equipped with full patient health context, assist in making informed decisions, ensuring doctors ask the right questions. AI scribes, such as Nuance's DAX Copilot and DeepScribe, automatically generate clinical notes, freeing physicians to focus on critical aspects of patient care.
Medical Training and Data Literacy
Data literacy is integral to modern medicine, encompassing research, insurance, and clinical operations. Medical professionals increasingly require knowledge of data analytics and programming languages like R and Python. Nate Verhagen’s Chat Clinic simulates patient interactions, helping students bridge the gap between theoretical knowledge and practical application.
Global Healthcare Systems and AI Adoption
Centralization vs. Decentralization
-
Decentralized Systems:
- Example: United States
- Characteristics: Privately owned, multiple electronic health record (EHR) systems, competitive market forces.
- Benefits: Encourages innovation through competition.
- Challenges: Fragmented data, lack of standardized data collection, and logistical barriers.
-
Centralized Systems:
- Example: United Kingdom (NHS)
- Characteristics: Single, publicly funded system, centralized data control.
- Benefits: Easier implementation of standardized data collection and sharing, comprehensive national databases.
- Challenges: Bureaucratic hurdles, slower innovation due to lack of competition.
Capturing and Utilizing Data for AI
Centralized Data Systems:
- Examples: National COVID Cohort Collaborative (N3C) in the U.S., UK Biobank.
- Benefits: Facilitates large-scale data collection and sharing, essential for developing robust AI models.
- Role in COVID-19: Enabled rapid health data aggregation and analysis, informing public health decisions.
Decentralized Data Systems:
- Benefits: Promotes innovation through competition, diverse approaches.
- Challenges: Difficulty in aggregating data from multiple sources, interoperability issues.
Public Healthcare Systems and Market Forces
Public healthcare systems might lack direct competitive pressures but can benefit from centralized initiatives driving AI adoption. Centralization enables efficient data integration and resource allocation for AI development.
Differences in AI Adoption: U.S. vs. U.K.
- United States: Driven by private sector innovation, multiple EHR systems, high investment in health tech startups. Example: University of Wisconsin-Madison's proximity to EPIC.
- United Kingdom: Centralized NHS approach facilitates national initiatives. Challenges include bureaucratic processes but potential for uniform data standards.
Impact on Research and Patient Care
Centralized Data Systems:
- Example: UK Biobank
- Benefits: Comprehensive datasets, easier longitudinal studies, large-scale AI training models.
- Impact: Enhanced research capability, improved patient care through data-driven insights.
Decentralized Data Systems:
- Benefits: Encourages diverse research approaches, fosters innovation.
- Impact: Potential for fragmented research efforts, challenges in data standardization and integration.
AI's Role During COVID-19
AI facilitated the rapid analysis of vast health data during the pandemic, highlighting the importance of centralized data systems in managing health crises effectively.
The Ethics and Regulation of AI in Healthcare
Data Privacy and Security
Ensuring compliance with regulations like HIPAA in the U.S. is crucial to protect patient information. Robust security measures must prevent data breaches and unauthorized access.
Bias and Fairness
AI systems can inherit biases from training data, leading to disparities in healthcare outcomes. Transparent and explainable AI models are essential to address biases and ensure equitable care.
Regulatory Landscape
The FDA's approval of 171 AI devices in 2023 signals growing acceptance. However, regulatory bodies must refine guidelines to ensure safe and effective AI use in healthcare.
The Future of AI in Healthcare
Personalized Healthcare
AI enables personalized healthcare by analyzing genetic data, lifestyle, and environmental factors, tailoring treatments to individual needs. This approach improves outcomes and reduces adverse effects.
Empathetic AI Agents
Collaborations like Hippocratic AI and NVIDIA aim to develop empathetic AI healthcare agents that interact with patients and build emotional connections, potentially outperforming human nurses in certain tasks.
AI Digital Twins
Digital twins, virtual replicas integrating biometric data, model and predict health outcomes. They empower individuals to make informed decisions, prevent diseases, and personalize health management.
Overcoming Barriers to AI Adoption
Resistance to Change:
- Education and training programs are essential to help healthcare professionals understand AI's benefits.
Integration with Existing Systems:
- Ensuring compatibility and seamless data flow between AI tools and EHRs is crucial.
Trust and Explainability:
- AI models must be explainable to build trust among healthcare professionals.
Conclusion
AI is poised to revolutionize healthcare by improving diagnostic accuracy, reducing administrative burdens, and enabling personalized treatments. While challenges remain, such as ensuring data privacy and overcoming resistance to change, the potential benefits of AI in healthcare are huge. By continuing to innovate and address these challenges, we can create a future where AI plays a central role in delivering high-quality, efficient, and personalized healthcare.
For more insights into the role of AI in healthcare, tune into our podcast episode featuring Leo and Nate, where we go deeper into these topics and explore the future of healthcare innovation!